AI: Science Fiction or Fact?
April 28, 2022
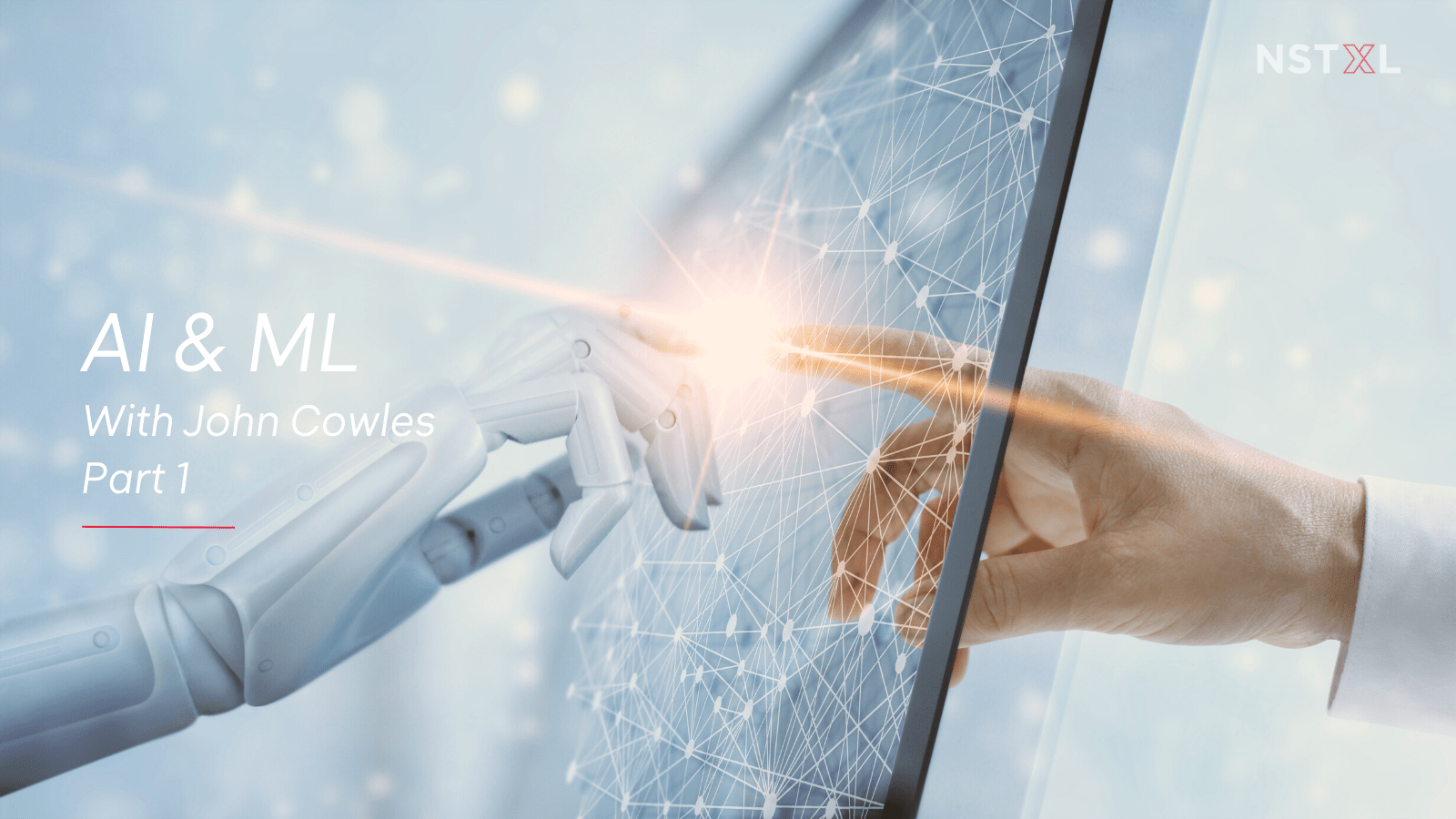
Written By: John Cowles, Senior Director of Engineering and Technology at Analog Devices
The Basics of AI & ML
Technologists love to introduce catchy acronyms and cryptic language into our vernacular to describe new ideas, trends, formats, or products. We might casually invoke Cloud Computing, IoT, and HTML without knowing exactly what they are. More importantly than just creating new language, technology has always promised to solve key generational challenges. Automation in factories meant that workers no longer needed to toil in dangerous conditions to survive. Food production efficiency averted the worst fears that global hunger would lead to war and mass extermination. Mobile phones have freed us to get more accomplished in our daily lives. It is easy to fall into the trap of expecting technology to solve all problems without any consequences. Each of the liberating advances mentioned above has had unexpected and unintended consequences downstream. Some of these include job insecurity, susceptibility of mega-scale monocultures to disease, and an expectation to be reachable 24-7, respectively.
Machine Learning (ML) and Artificial Intelligence (AI) are quoted everywhere today. Along with many other concepts, they have unjustly been touted as the solutions to all our problems. It took years until today’s computing power, memory storage, and cloud/internet services caught up to the needs of useful and deployable learning algorithms. Today we host language processing and image recognition on our smartphones and take weather, traffic, and stock predictions for granted.
What is AI?
What are practical and applicable definitions of AI and ML? Are they one and the same? AI, like natural (human) intelligence, is easier to recognize than to define. AI is attributed to machines that can learn and solve problems. They can also perceive and react to their environments, and even interact naturally with humans. Specific examples are image recognition and classification, autonomous driving, web searching, recommendation systems, gaming, chatbots, and many more. Historically ML was one of many sub-topics within the broader area of AI. ML is the discipline of learning patterns and insights from data to predict the future based on a set of observed features. Increasingly, ML is not only predicting but also acting on the insights. The parallels to our own development from childhood based on experience, learning, and rewards are amazing. This will become clearer once we discuss Deep Learning and Neural Networks.
Unintended Consequences
We don’t need to contemplate the unintended consequences of AI and ML as they continue to impact our lives. Sci-Fi and Hollywood have already anticipated plenty of dark scenarios once machines gain consciousness. Recall HAL9000’s (Heuristic Algorithmic Computer) in the movie 2001 Space Odyssey, the self-aware Skynet network depicted in The Terminator series, or the AI that escaped control in the Replicants of Blade Runner. In opposition, AI is already helping our daily routines by optimizing driving times, deciding what products best fit our needs, and helping scientists synthesize better vaccines. AI is just another tool like the wheel, factory assembly lines, and computers of the past but with a much broader impact across all aspects of our lives.
Where We Go From Here
With these juxtaposed themes in the background, we will take a more pragmatic journey in the next installments starting with a review of the taxonomy and types of learning algorithms that are popular today. These installments will come once per quarter and will continually dive deeper into the world of AI as they go. With this foundation, we will discuss the kinds of problems that are reasonably addressed by AI/ML, including those that are already deployed. I am excited to take this journey and learn as we go about AI’s place in the future of our society.